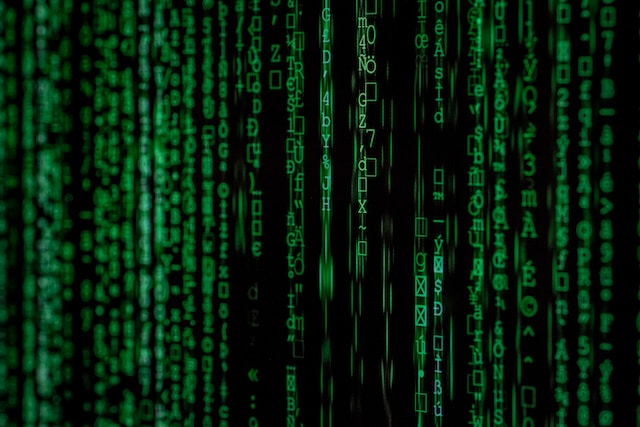
https://unsplash.com/photos/matrix-movie-still-iar-afB0QQw?utm_content=creditShareLink&utm_medium=referral&utm_source=unsplash
Table of Contents
Introduction
Integer conversion between bases 10 and 2 (binary) is necessary and extensively used in many areas of science and engineering. Digital communications, for example, are intrinsically binary, which implies that any analog signal upstream of transmission must be, to use an inappropriate mathematical term, “base-changed”.
In artificial intelligence and machine learning, many algorithms require a bidirectional conversion between the two bases. For example, genetic algorithms (GA) have traditionally been considered to work best on genotypes with low cardinality encodings, so the problem parameters are normally encoded as binary strings whose concatenation generates the complete genotype of each solution or chromosome. There are exceptions to this way of proceeding (some GAs directly use the parameters in the decimal representation in the construction of the chromosome), but we can say that most genetic algorithms are designed this way.
Problems Related to Decimal-Binary Conversion
However, within certain disciplines, converting an integer from decimal to binary representation in a strictly positional manner is problematic. Let’s consider the decimal and binary representations of the first 10 integers:
Decimal | Binary |
---|---|
0 | 0000 |
1 | 0001 |
2 | 0010 |
3 | 0011 |
4 | 0100 |
5 | 0101 |
6 | 0110 |
7 | 0111 |
8 | 1000 |
9 | 1001 |
10 | 1010 |
As can be seen, the difference of one unit in the decimal representation is not accompanied in general by the variation of a single bit in the binary representation. This effect is particularly evident when the transition from one decimal integer to the next requires an additional bit in base 2, as happens between 1 and 2, 3 and 4, or 7 and 8. In this case, all the bits in the binary representation change.
In the case of genetic algorithms, this behavior represents an obstacle in the learning process, as it introduces discontinuities in decoding and causes a misalignment between genotype and phenotype.
Even before the advent of artificial intelligence and machine learning, the problem was felt within certain electromechanical procedures. In 1953 Frank Gray of Bell Laboratories solved the problem by designing and patenting the code that bears his name. Gray’s code eliminates the issues mentioned above because two consecutive integers in the decimal representation generate two integers in the binary representation that differ in a single bit.
In the case of GAs, Gray’s code also mitigates other difficulties, such as the so-called “Hamming walls”. These are points at which it becomes rare or highly unlikely that the GA will mutate in exactly the right way to produce the next step in fitness. Within digital communications, Gray’s code facilitates the correction of errors in transmissions.
The encoding/decoding between the decimal representation and the Gray encoding does not occur directly but requires an intermediate binary conversion:
Decimal → Binary → Gray
Gray → Binary → Decimal
Conversion from Binary to Gray Code
The most significant bit of the number in the binary representation or MSB (Most Significant Bit), which corresponds to the leftmost bit in a big-endian architecture, remains unchanged. Subsequent bits in the Gray encoding are obtained by applying the XOR operator between each bit and the next bit of the original binary representation.
In C code:
void bin2gray(char *B, char *G) { char *ptrB = B, *ptrG = G; *ptrG++ = *ptrB++; while (*ptrB) { if (*(ptrB - 1) != *ptrB) { *ptrG++ = '1'; } else { *ptrG++ = '0'; } ptrB++; } *ptrG = '\0'; }
The variables B and G are two strings of characters corresponding respectively to the starting binary representation and the generated Gray code.
Conversion from Gray Code to Binary
The MSB bit remains unchanged. Then the XOR operator is applied between each bit of the binary representation and the next bit of the gray code. In C code:
void gray2bin(char *G, char *B) { char * ptrB = B, *ptrG = G; *ptrB++ = *ptrG++; while (*ptrG) { if (*(ptrB - 1) != *ptrG) { *ptrB++ = '1'; } else { *ptrB++ = '0'; } ptrG++; } *ptrB = '\0'; }
Conclusion
Converting integers between decimal and binary representations in a strictly positional manner is not optimal within certain scientific problems, and can give rise to aberrations that hinder the way computation algorithms work.
One of the main issues concerns the fact that the binary coding obtained starting from an integer in decimal representation gives rise to numbers that differ in more than one bit between two consecutive integers. Gray coding solves this problem, allowing increased performance within numerical procedures involving conversions between bases 10 and 2.