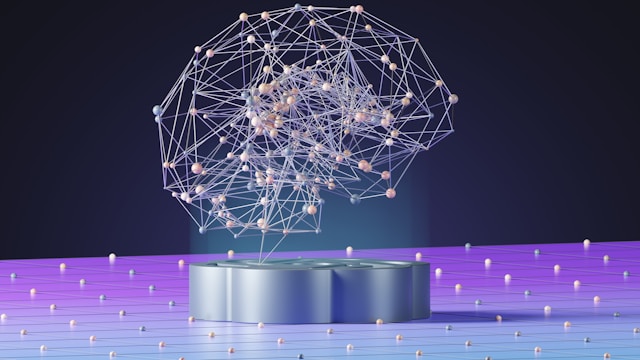
https://unsplash.com/photos/an-abstract-image-of-a-sphere-with-dots-and-lines-nGoCBxiaRO0?utm_content=creditShareLink&utm_medium=referral&utm_source=unsplash
Backpropagation is a generalization of the Delta rule to be able to take into account non-linear functions.
Suppose a differentiable function of the input vector
$$y_{k}^{p}=f(s_{k}^{p})$$
where \(k\) represents the \(k\)-th unit of the network and \(p\) the \(p\)-th pattern. \(f (s_ {k} ^ {p})\) is called activation function, and depends on a linear combination of the weights and outputs of the units of the previous layer of the network, which become the inputs for the unit \(k\). Of course, \(y_ {k} ^ {p}\) is the output generated by the network for the \(k\)-th unit, according to the functio \(s_ {k} ^ {p}\)
$$s_{k}^{p}=\sum_{j}w_{jk}y_{j}^{p}+\theta_{k}$$
The summation extends to all units of the level immediately preceding the one in which unit \(k\) is located
$$j=k-1$$
\(\theta_ {k}\) is the bias term. It is associated to the layer in which the unit \(k\) is located and not to the layer \(j\).
We want to find the weight variation rule that causes a decrease in the error
$$\Delta_{p}w_{jk}=-\gamma\frac{\partial E^{p}}{\partial w_{jk}}$$
being \(\gamma\) a constant of proportionality called learning rate. Using squared error as measure of error
$$E^{p}=\frac{1}{2}\sum_{o=1}^{N_{o}}(t_{o}^{p}-y_{o}^{p})^{2}$$
where the error is measured on all output units as the difference between the value generated by the network, \(y_ {o} ^ {p}\), and the target value \(t_ {o} ^ {p}\). The \(\frac {factor 1} {2}\) is arbitrary and is introduced to be canceled with the quadratic exponent during derivation. This allows a slightly more simplified final expression.
The total error is the sum of the quadratic error of the set of patterns
$$E=\sum_{p}E^{p}$$
According to the chain rule
$$\frac{\partial E^{P}}{\partial w_{jk}}=\frac{\partial E^{p}}{\partial s_{k}^{p}}\frac{\partial s_{k}^{p}}{\partial w_{jk}}$$
from the equation which connects the output of one unit and the weighted sum of the units of the previous layer, we get
$$\frac{\partial s_{k}^{p}}{\partial w_{jk}}=y_{j}^{p}$$
We define the other derivative in the equation as
$$\frac{\partial E^{p}}{\partial s_{k}^{p}}=-\delta_{k}^{p}$$
and we get
$$\Delta_{p}w_{jk}=\gamma\delta_{k}^{p}y_{j}^{p}$$
\(\delta_ {k} ^ {p}\) exists for every unit on the network. It is possible to compute \(\delta_ {k} ^ {p}\) through a recursive procedure that propagates the errors back through the network, starting from the output units where the error is computable since the target value is known.
To calculate \( \delta_ {k} ^ {p}\) we divide the derivative of the equation, using the chain rule, into two contributions: one that reflects changes with the variation of the input and another that reflects changes with the variation of the output
$$\delta_{k}^{p}=-\frac{\partial E^{p}}{\partial s_{k}^{p}}=-\frac{\partial E^{p}}{\partial y_{k}^{p}}\frac{\partial y_{k}^{p}}{\partial s_{k}^{p}}$$
and from the definition of activation function
$$\frac{\partial y_{k}^{p}}{\partial s_{k}^{p}}=f'(s_{k}^{p})$$
To calculate \(\frac{\partial E^{p}}{\partial y_{k}^{p}}\), we consider two cases.
1 \(k\) is a network output unit
$$\frac{\partial E^{p}}{\partial y_{o}^{p}}=\frac{\partial}{\partial y_{o}^{p}}\left[\frac{1}{2}\sum_{o=1}^{N_{o}}(t_{o}^{p}-y_{o}^{p})^{2}\right]=-(t_{o}^{p}-y_{o}^{p})$$
and therefore
$$\delta_{o}^{p}=(t_{o}^{p}-y_{o}^{p})f'(s_{o}^{p})$$
2 \(k\) is a hidden unit
For a hidden unit \(k = h\) we do not know the contribution of that unit to the output of the network. However, we can write the error as a function of the input of the hidden units to the output units
$$E^{p}=f(s_{1}^{p},s_{2}^{p},\ldots,s_{j}^{p},\ldots)$$
and using the chain rule
$$\begin{array}{rcl} \frac{\partial E^{p}}{\partial y_{h}^{p}} & = & \sum_{o=1}^{N_{o}}\frac{\partial E^{p}}{\partial s_{o}^{p}}\frac{\partial s_{o}^{p}}{\partial y_{h}^{p}}\\ & = & \sum_{o=1}^{N_{o}}\frac{\partial E^{p}}{\partial s_{o}^{p}}\frac{\partial}{\partial y_{h}^{p}}\left(\sum_{j=1}^{N_{h}}w_{jo}y_{j}^{p}\right)\\ & = & \sum_{o=1}^{N_{o}}\frac{\partial E^{p}}{\partial s_{o}^{p}}w_{ho}\\ & = & -\sum_{o=1}^{N_{o}}\delta_{o}^{p}w_{ho} \end{array}$$
we obtain
$$s_{h}^{p}=f'(s_{h}^{p})\sum_{o=1}^{N_{o}}\delta_{o}^{p}w_{ho}$$